The Personalized Privacy Assistant Project*
The Internet of Things (IoT) and Big Data are making it impractical for people to keep up with the many different ways in which their data can potentially be collected and processed. What is needed is a new, more scalable paradigm that empowers users to regain appropriate control over their data. We envision personalized privacy assistants as intelligent agents capable of learning the privacy preferences of their users over time, semi-automatically configuring many settings, and making many privacy decisions on their behalf. Through targeted interactions, privacy assistants will help their users better appreciate the ramifications associated with the processing of their data, and empower them to control such processing in an intuitive and effective manner. This includes selectively alerting users about practices they may not feel comfortable with, confirming with users privacy settings the assistants are not sure how to configure, refining models of their user’s preferences over time, and occasionally nudging users to carefully (re)consider the implications of some of their privacy decisions. Ultimately, these assistants will learn our preferences and help us more effectively manage our privacy settings across a wide range of devices and environments without the need for frequent interruptions.
This video is hosted on YouTube. See YouTube's Privacy Policy here.
* US Patent 10,956,586, US Patent 11,768,949, and additional USPTO and EPO Patents Pending
Our project combines multiple research strands, each focusing on complementary research questions and elements of functionality. Our work is driven by user-centered design processes that translate personal privacy preference models, transparency mechanisms and dialog primitives into personalized privacy assistant functionality. We employ a wide range of quantitative and qualitative research methods, inlcuding data mining, machine learning, lab experiments, surveys and interviews, to evaluate and refine our functionality.
Learn more about our research by topic:
We are developing a privacy infrastructure for the increasingly ubiquitous Internet of Things (IoT). This infrastructure enables privacy assistants to discover relevant IoT resources, relevant elements of their privacy policies and any available privacy settings. Learn more about this infrastructure on our IoT page.
Selected Publications:
- A. Das, M. Degeling, D. Smullen, and N. Sadeh, Personalized Privacy Assistants for the Internet of Things, 2018 IEEE Pervasive Computing: Special Issue - Securing the IoT, April 2018
- A. Das, M. Degeling, X. Wang, J. Wang, N. Sadeh and M. Satyanarayanan, Assisting Users in a World Full of Cameras: A Privacy-aware Infrastructure for Computer Vision Applications, 2017 IEEE Conference on Computer Vision and Pattern Recognition Workshops (CVPRW), July 2017
We are developing user-oriented machine learning techniques to capture people’s privacy preferences and expectations. These models are used to help users manage an otherwise unmanageable number of privacy decisions. This includes recommending or semi-automating the configuration of many privacy settings for individual users.
Selected Publications:
- P. Emami-Naeini, S. Bhagavatula, H. Habib, M. Degeling, L. Bauer, L. Cranor, and N. Sadeh, Privacy Expectations and Preferences in an IoT World, Symposium on Usable Privacy and Security (SOUPS '17), July 2017
- B. Liu, M.S. Andersen, F. Schaub, H. Almuhimedi, S. Zhang, N. Sadeh, A. Acquisti, and Y. Agarwal, Follow My Recommendations: A Personalized Assistant for Mobile App Permissions, Symposium on Usable Privacy and Security (SOUPS '16), June 2016
- J. Lin, B. Liu, N. Sadeh, and J.I. Hong, Modeling Users’ Mobile App Privacy Preferences: Restoring Usability in a Sea of Permission Settings, 2014 ACM Symposium on Usable Security and Privacy (SOUPS '2014), July 2014.
- B. Liu, J. Lin, N. Sadeh, Reconciling Mobile App Privacy and Usability on Smartphones: Could User Privacy Profiles Help?, 23rd Interntional Conference on the World Wide Web (WWW 2014).
- A. Sinha, Y. Li, and L. Bauer, What you want is not what you get: Predicting sharing policies for text-based content on Facebook, In Proc. AISec, 2013.
- J. Cranshaw, J. Mugan, N. Sadeh, User-Controllable Learning of Location Privacy Policies with Gaussian Mixture Models, In Proc. 25th AAAI Conference on Artificial Intelligence, August 2011.
- J. Mugan, T. Sharma, N. Sadeh, Understandable Learning of Privacy Preferences through Default Personas and Suggestions Carnegie Mellon University's School of Computer Science Technical Report CMU-ISR-11-112, 2011.
We are exploring the merits of different modes of interaction, different interaction primitives and different interaction styles with the user. As we move towards Internet of Things scenarios, Personalized Privacy Assistants will have to be increasingly parsimonious and effective in the way in which they interact with their users. This includes being able to accommodate a wide range of contextual factors that impact the availability and effectiveness of different forms of communication with the user. This also includes studying the impact of different solutions on user privacy decision making and more generally on their behavior. What does it take to get a user’s attention? How much information is too much? When is the best time to interact with the user? What mode of interaction is most effective in a given context? How does one nudge users to carefully weigh privacy-utility tradeoffs associated with their decisions? And more.
Selected Publications:
- J. Gluck, F. Schaub, A. Friedman, H. Habib, N. Sadeh, L.F. Cranor, and Y. Agarwal, How Short is Too Short? Implications of Length and Framing on the Effectiveness of Privacy Notices, Symposium on Usable Privacy and Security (SOUPS '16), June 2016
- F. Schaub, R. Balebako, A. Durity, L. Cranor, Design Space for Effective Privacy Notices, Symposium on Usable Privacy and Security (SOUPS '15), July 2015
- H. Almuhimedi, F. Schaub, N. Sadeh, Y. Agarwal, A. Acquisti, I. Adjerid, J. Gluck, L. Cranor, Your Location Has Been Shared 5398 Times! A Field Study on Mobile Privacy Nudges, in Proc. CHI, 2015.
- Y. Wang, P.G. Leon, A. Acquisti, L.F. Cranor, A. Forget, and N. Sadeh, A Field Trial of Privacy Nudges for Facebook, in Proc. 32nd annual SIGCHI Conference on Human Factors in Computing Systems, CHI2014. April, 2014.
- S. Wilson, J. Cranshaw, N. Sadeh, A. Acquisti, L. Cranor, J. Springfield, Sae Young Jeong, Arun Balasubramanian, Privacy Manipulation and Acclimation in a Location Sharing Application, in Proc. of the 15th ACM International Conference on Ubiquitous Computing (Ubicomp2013), Zurich, Switzerland, Sept. 2013.
We are developing transparency mechanisms for big data systems to inform users about data use practices of data holders. This includes identifying what data holders can infer from the data they collect and how they use the results. This analysis can also be used to help people better appreciate the ramifications of their privacy decisions.
Selected Publications:
- S. Zimmeck, Z. Wang, L. Zou, R. Iyengar, B. Liu, F. Schaub, S. Wilson, N. Sadeh, S.M. Bellovin, J.R. Reidenberg, Automated Analysis of Privacy Requirements for Mobile Apps, in AAAI Fall Symposium on Privacy and Language Technologies, Nov 2016.
- Amit Datta, Michael Carl Tschantz, Anupam Datta Automated Experiments on Ad Privacy Settings: A Tale of Opacity, Choice, and Discrimination, in Proc. Privacy Enhancing Technologies Symposium, July 2015.
- Michael Carl Tschantz, Amit Datta, Anupam Datta, Jeannette M. Wing, A Methodology for Information Flow Experiments, in Proc. 28th IEEE Computer Security Foundations Symposium, July 2015.
We are developing an architecture and elements of infrastructure to support the deployment of personalized privacy assistants across different mobile and Internet of Things (IoT) scenarios. This includes the identification of an extensible collection of privacy constructs that can be used by IoT resource owners to describe the data collection, use and sharing practices associated with these resources (e.g. sensors, applictions, services) in a machine readable manner. These primitives can then be interpreted by Personalized Privacy Assistants and selectively communicated to their users.
Selected References:
- Gandon, F. and Sadeh, N., Semantic Web Technologies to Reconcile Privacy and Context Awareness, Journal of Web Semantics. Vol. 1, No. 3, 2004.
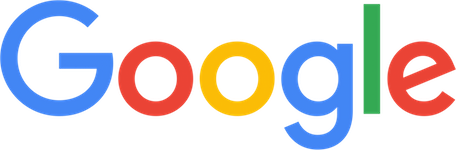
Google Web of Things Expedition
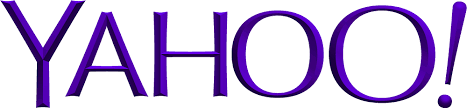